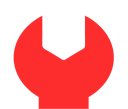
Our site is coming soon
We are doing some maintenance on our site. It won't take long, we promise. Come back and visit us again in a few days. Thank you for your patience!
We are doing some maintenance on our site. It won't take long, we promise. Come back and visit us again in a few days. Thank you for your patience!